Confidence Intervals
-
Point Estimators
and Confidence Intervals
Suppose that you are asked to determine the mean (i.e. average) age of
CSUS students during the current semester. The population, all CSUS
students consists of approximately 20,000 individuals. To obtain the
age of every student would be time consuming and costly. However, you
could estimate the mean age by taking a small random sample of current
students, get the age of each sampled student, and average these
numbers. For example, a random sample of 10 students might produce the
following collection of ages: 19, 21, 30, 32, 22, 23, 26, 18, 20, and
21. The average of these numbers, 23.2 is an estimate of the mean age
of all students. A single number estimate is called a point
estimate.
There are a couple of questions connected with this point
estimate. First, is the mean of the sample the best point estimate
of the population mean. Perhaps there are ways, other than
averaging, to combine the sampled ages to produce a better estimate of the
population mean. Statisticians have developed some measures of
quality for point estimators. One of them is called 'unbiasedness.'
An estimator of a population parameter if when all possible samples are
selected from the population and the estimator is computed for each
sample, the average of these estimators equals the population parameter
that you are trying to determine. In statistical terms, and unbiased
estimator is an estimator whose expected value equals the population
parameter. In symbols, an estimator of a parameter
of a population is denoted by
(read 'theta hat'). An unbiased estimator satisfies the
condition .
It can be shown that the sample mean is an unbiased estimator of the
population mean.
Estimators of population parameters vary from sample to sample. So,
a
second desirable property of a point estimator is that these estimators
have minimal variation, that is, minimal variance. It turns out that among all unbiased estimators of the
population mean for a sample of fixed size n, the sample mean has the
smallest variance. So, given the 10 data values, the average of
them, 23.2, is the best point estimator of the population mean age of CSUS
students in the sense that it is unbiased and has the smallest variance.
In summary, a point estimate of a population parameter is a single number
based on the sample used to estimate a population parameter.
Given a single number
point estimate of a population parameter like the point estimate 23.2 for
the mean age of all CSUS students, you might wonder how close this number
is to the population mean. It is unlikely that the estimate, 23.2,
is exactly equal to the population mean. If it isn't equal, is it
off by 0.2 years? by 1 year? by 10 years? If you are only given the
single number estimate, you have no information concerning the 'closeness'
of the estimate to the parameter. Confidence intervals are numerical
intervals in which the population mean might
lie. Together with the interval, a measure of certainty that the
parameter lies within the interval is given. For example, in the
example of the mean age of CSUS students, a confidence interval would be
given in the following form: 'you are 95% confident that the mean age of
CSUS students lies in the interval from 21.2 years to 25.2 years.'
These two items, the confidence level, and the length of the confidence
interval give you much more information than a single number.
In the following sections the meaning of the term confidence level, and
the processes used to compute the endpoints of confidence intervals are
shown for several population parameters.
-
Confidence Interval for a Population Mean
-
Population
Standard Deviation Known
In this section a confidence interval for the population mean when the
population standard deviation is know is developed. You might ask, why
if the population mean is unknown, would the population standard deviation
be known? In many cases the population standard deviation is unknown,
and confidence intervals will be developed for that situation later.
Also, in many situations you may not know the population mean but you do
know the population standard deviation. These are the situations that
are considered here.
From your work with the sampling distribution of sample means, you know
that the sampling distribution of sample means is approximately normal, its
mean is the mean of the population from which samples are selected, and its
standard deviation is the population standard deviation divided by the
square root of the sample size. If a random sample of size n is
selected and the mean computed, the following statement is true:
A confidence interval with confidence level (1- )100% is
determined as follows: (1) find two z-values with the property that between
them the probability is 1- . By symmetry of the normal density
function, one of the z-values will be the negative of the other
z-value. (2) Put each of these z-values in the formula shown above and
solve the equation for .
(3) The interval formed by these two solutions is the 1-alpha confidence
interval for .
The formula for the confidence interval can be written
where is
the z-value with probability to
the right of it.
When a confidence interval based on a single sample is
computed, this confidence interval might or might not contain the population
mean. If the confidence interval contains the mean, it is called
'good', and if the confidence interval doesn't contain the mean, it is
called 'bad.' Since, in practice, you won't know the population mean,
you won't know whether the single confidence interval that you compute is
'good' or 'bad'. However, the following demonstration shows that in
the long run (1- )100%
of the confidence intervals will be good. A
link to a Hyperstat Online page from Rice University that
demonstrates the meaning of confidence level is found here.
-
Population
Standard Deviation Unknown
To find a (1- )100%
confidence interval for
when the population standard deviation is unknown, you would like to replace
with s, the
sample standard deviation in the formula

However, if you replace
with s,

doesn't have a standard normal distribution. If it
did, we could find two z-values such that the probability between them is
(1- ), put each of
them on the left side of the last expression, and solve for ,
thus producing the desired confidence interval.
As you take all possible random samples for size n from a
population, what kind of probability distribution does have?
For a general population and small sample size (n<30), there is no
answer. If n is 30 or more the distribution is close to a standard
normal distribution, but for n<30, the only case in which the probability
distribution is known is the case in which the population from which samples
are selected is normally distributed. In that case the statistician
Gossett described the probability distribution as a 'Student's
t-distribution with n-1 degrees of freedom'. What is the Student's
t-distribution with n-1 degrees of freedom? The following graph shows
a standard normal distribution in black, a t-distribution with 5 degrees of
freedom in blue, and a 5-distribution with 20 degrees of freedom in red.
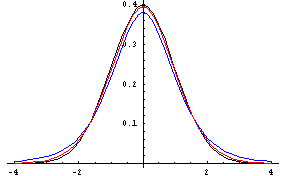
You can see that the t-distributions have slightly greater
variability than the standard normal distribution. Also, as degrees of
freedom increase, the t-distribution curve gets closer to the standard
normal curve. You can use the following page to find any
t-distribution probabilities. Link
to a calculator page from the UCLA Statistics Department that will allow you
to find the cdf (or pdf) for any Student's t distribution (Called the
Student Distribution).
A formula for a (1- )100%
confidence interval for
when is unknown
is

where t has n-1 degrees of freedom.
-
Confidence Interval for a Population Proportion
Confidence intervals
for population proportions appear almost daily in newspapers, in magazine
articles, and on radio and television broadcasts. They are used to
predict the proportion of a population with a certain
characteristic. For example, the proportion of a population with
brown hair, the proportion of a population favoring the death penalty, the
proportion of the population that smokes, etc. A most interesting
application of confidence intervals is prediction of election
outcomes. Professor Richard Lowry of Vassar College has written a
wonderful web page on election
polling and the 2000 presidential election. The proportion of the
population possessing the characteristic of interest is denoted by
p. Since the population is often very large, in order to predict p,
a random sample of size n is selected from the population. If X is
the number of elements of the sample with the characteristic of interest,
X can, in most cases, be considered to be a binomial random variable with
parameters n and p. The sample proportion with the characteristic of
interest is denoted by .
Then =X/n.
Since
is an average, the central limit theorem implies that for large n, approximately
normally distributed with mean E[X/n]=(1/n)E[X]=(1/n)(np)=p and Var[X/n]=(1/n2)Var[X]=(1/n2)(npq)=pq/n.
This means
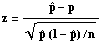
has a normal distribution. To find a (1- )100%
confidence level confidence interval find the appropriate z-values, put
them in the last equation, and solve for p. You will get
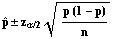
There is a problem with this expression. Since you
are finding a confidence interval for p, you don't have a value for
p. This problem can be overcome by using the estimator in
place of the unknown p. This results in the following confidence
interval formula
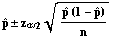
-
Confidence Interval for a Population
Variance
A (1- )100%
confidence level confidence interval for the population variance, 2,
can only be found when the population from which the sample is drawn is normally
distributed. In this case, you have seen that the quantity

has a Chi-Square distribution with n-1 degrees of freedom
where S2 is the sample variance computed by using the formula
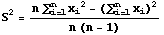
To find the confidence interval, use the table of the
Chi-Square distribution with n-1 degrees of freedom to find two Chi-Square
values such that the probability between them is 1- .
These two values are denoted by
and . By
replacing the left hand side of the expression for Chi-Square shown above by
each of these quantities and solving in each case for 2
you get the confidence interval from
to

To find a confidence interval for simply
take the square root of the endpoints of the confidence interval for the
variance.
-
Confidence Interval for Difference of
Proportions
|